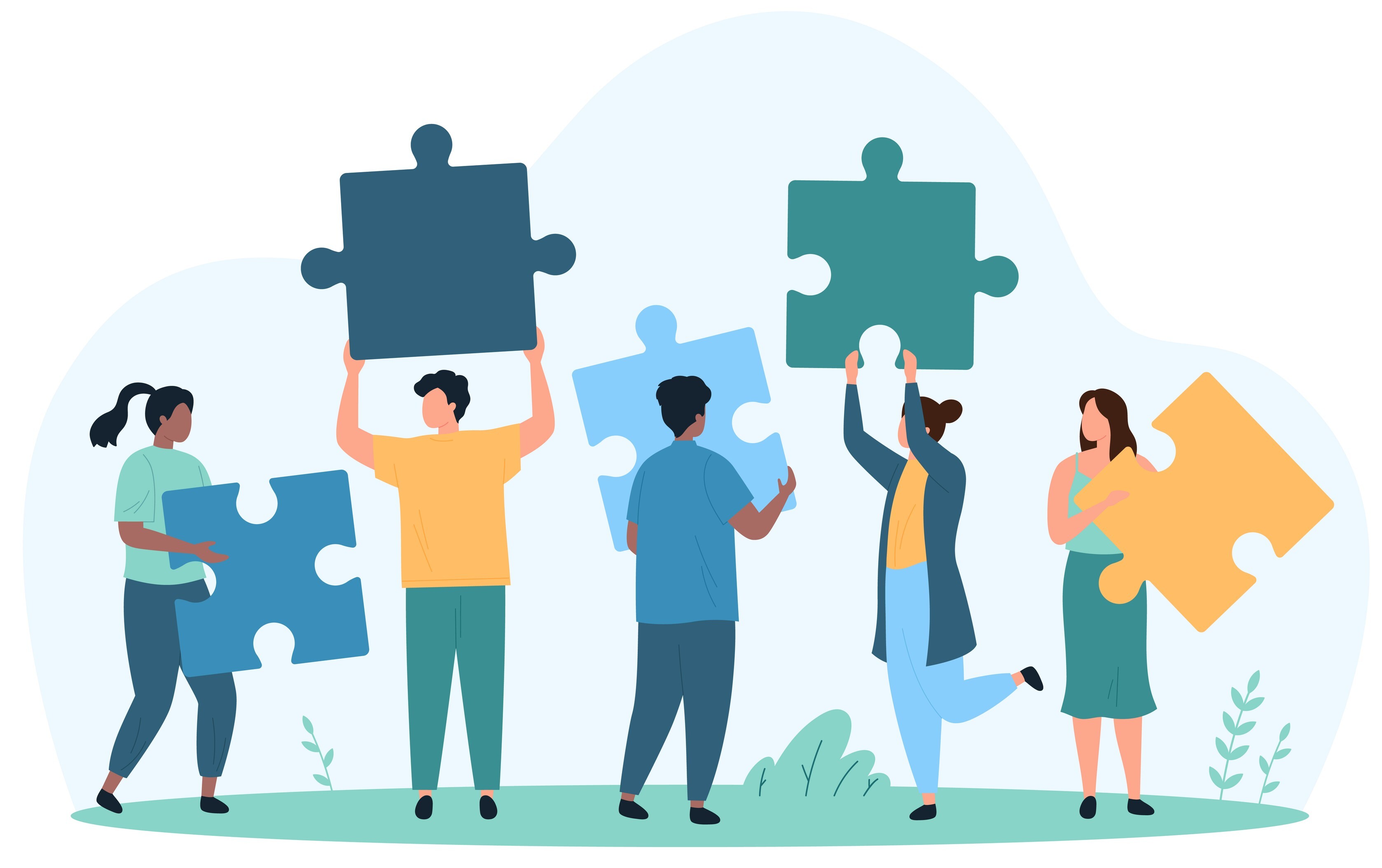
Become a member
Membership of IUFRO is open to any institution concerned with the promotion, support or conduct of research related to forests, trees and forest products.
Quantifying tree mortality at global scale remains a major unsolved challenge. Earth observation satellites, such as the Copernicus Sentinel fleet have sufficient resolution to map even scattered tree mortality patterns. Coupled with supervised machine learning, they present a promising avenue to map standing dead trees at global scale. To acquire data for training globally transferable machine learning models training data, we need a globally comprehensive reference dataset.
Here, we present the launch of the community-based deadtrees.earth database, compromising more than 1500 globally distributed centimeter-scale drone orthophotos of forests with delineated standing deadwood. This database acts as a foundation to a machine learning model ecosystem that leverages these drone orthophotos and labels as reference for satellite-based tree mortality mapping. Finally, we present a model that provides a yearly map, fractional cover of standing deadwood at 10 m resolution, across global ecosystems. These products will facilitate to reveal and understand global tree mortality dynamics across.
Speakers
Teja Kattenborn, Freiburg University
Clemens Mosig, Leipzig University